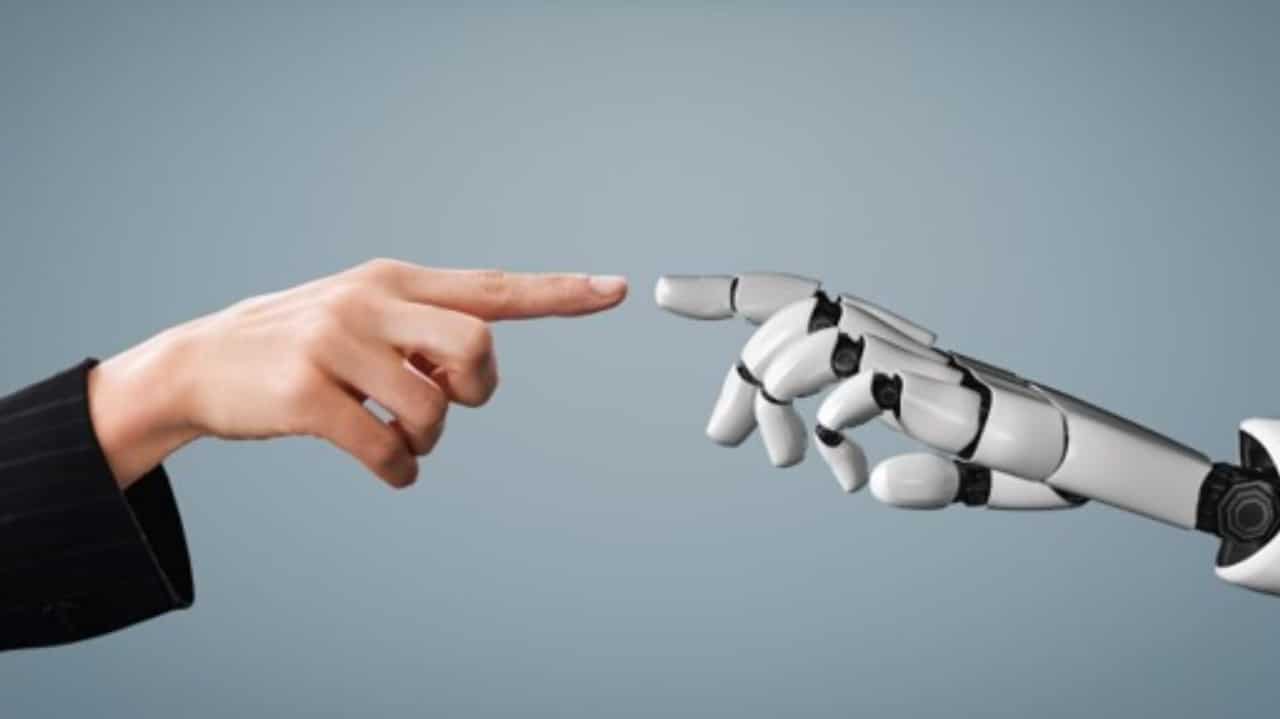
We are finally hitting the tipping point in the hype cycle of AI because the business world has figured out that training off public data is scalable and repeatable. It appears everything has changed, yet nothing has changed at the same time. Business leaders still need to be focused on the customer. Best practice still needs to be documented. Your mission has not changed.
How do leaders incorporate AI into their planning? What’s different about this process? Nothing. The real question is what aspects of their business are leaders now going to do faster and better with AI.
There is excitement about the possibilities of AI out there but also recognition of the disruption it creates.
According to IBM, 35% of businesses were already using AI in 2022, and a further 42% were considering adopting it. People are triangulating until they understand how AI works in practice before incorporating it into their operations. I believe once leaders have a solid plan for implementation and integration, optimism about the advantages of AI will really start to hit home.
Addressing the concerns of leaders
Right now, leaders appear divided over the potential of AI. Some are enthusiastic about the new capabilities to solve complex problems, while the hesitancy of others mirrors the uncertainty over what the future of AI will look like. Just as AI can sort meaningful patterns from irrelevant data, so can we filter the signal from a lot of the noise around generative AI in particular.
Often, leaders want to adopt but don’t know where to begin. For starters, deep learning and generative AI are not part of the use case of the typical enterprise. Instead, it is a matter of applying the normal prioritization process. If you are not constrained anymore by people and information, ask what areas would most benefit from more speed and personalization because that’s the takeaway of what this technology is offering.
Despite concerns about the costs of bringing in new partners and software, hitting that tipping point means every business has to have a strategy. Yet, for all the speed of AI’s apparent arrival, leaders can afford to be slow and thoughtful about implementation because no service provider is going to give you everything you want out of the box.
Why? Because some of their solutions will integrate open source with private data, and a period of using machine learning for customization is needed. In fact, the combination of public and private data is the most fertile ground for growth. This is the potential for transformation that will likely shift perspectives on AI.
What are the potential dangers of AI?
There’s no denying that adopting AI comes with risk. Leaders are right to have ethical concerns over machine learning using large data sets to entrench real-world bias and discrimination. There can also be a lack of explainability when AI is unsupervised. Those are the surprises when AI gets it wrong.
But training-data discrimination and the time it takes to break down and explain AI decisions only reiterate the need for human oversight. While the models will continue to evolve as more data becomes available, in the meantime finding the right partners can help businesses find the right balance between supervised and unsupervised use of AI.
As partners, we are not going to lead you straight to running AI completely autonomously — if we give you a code in the script, you will still have to look and see if it is right. Some of the other guardrails for the safe and ethical use of AI include leveraging open-source tools for bias mitigation, regularly inspecting for clean data, and documenting best practices.
Another of the main concerns is that AI tools can leave vulnerable systems open to data breaches, so it is crucial for leaders to manage and safeguard the data used to train AI models with updated cybersecurity tools and encryption. Again, none of this is a significant deviation from standard practice.
Lastly, many employees worry about job displacement, but automation creates opportunities for value-adding roles elsewhere. Reskilling and upskilling is a crucial component of rewarding staff for their adaptability and engaging them in the transition.
The two facets of transformation
There are two buckets to implementing AI if I am a company leader: What I want to do for customers and how to run my business more optimally. We are not reinventing the business model. In the Amazon world, whenever we learned something new, we would add the protocols for how we got it done to the documentation.
In the same vein, I suggest leaders engrain AI in the culture through communication, change management, and setting up employees’ expectations by incentivizing their participation. We are at the tipping point, and it is no longer a question of adoption but how to manage the transformation.