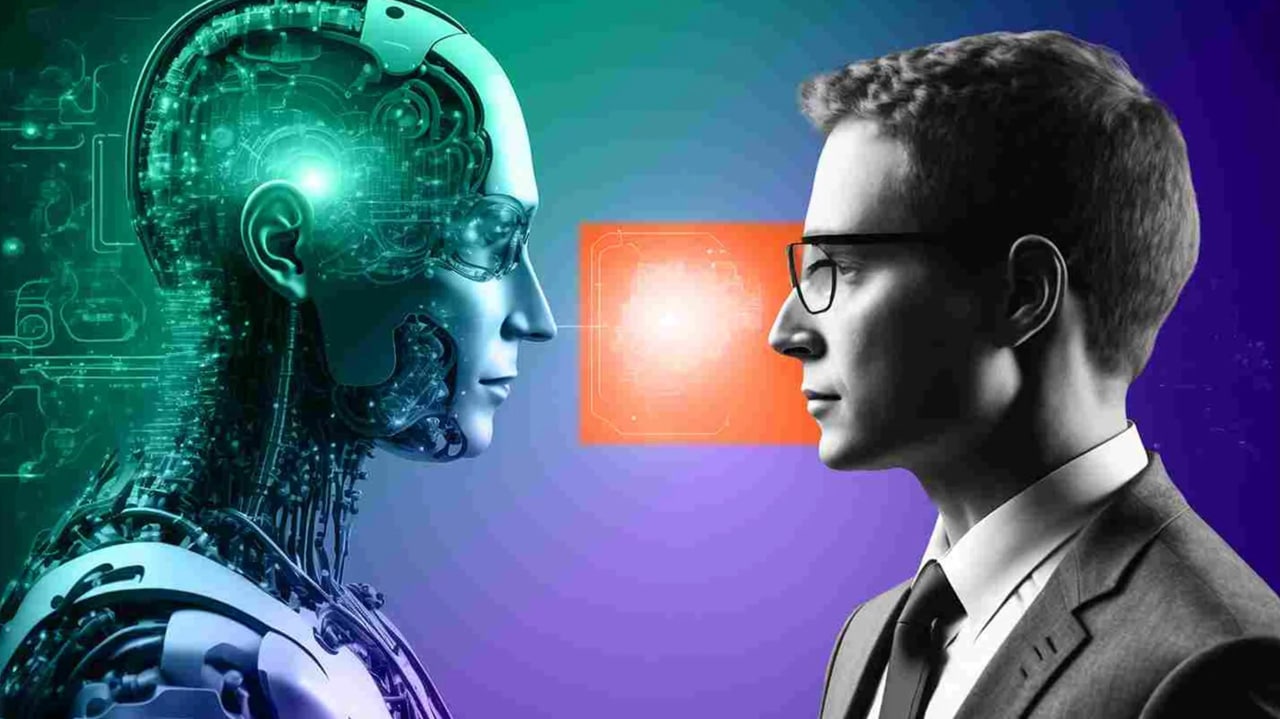
Despite all the noise around generative AI (GenAI), most businesses are not operating in new or dramatically different territories. They have not suddenly adopted a new mission based on AI. I see the shift this technology is triggering as analogous to the internet’s impact on retail. At the time, nobody had a business plan for physical retail that included online, but companies that put digital at the core of how they operated ended up benefiting the most.
Looking at the five stages of the Gartner Hype Cycle, which tracks how emerging technologies are adopted and used, I believe we are still at the beginning of the curve, where media and industry focus on applications. GenAI, which has attracted most of the press, may be supercharging the likes of Adobe’s graphic-design products, but the enterprise use case of AI at large is not as sophisticated.
In other words, the fundamentals aren’t changing: If you are a fraud detection company, you still have to detect anomalies and battle security, but now you can train and harness the power of machines to do it better and faster. To business leaders getting caught in the hype, I say: Slow down. Go back to your playbook and first principles as you incorporate AI into your culture and business model.
1. Identify The Right Areas For Investment
The one certainty in this space is that every business must have an AI strategy. However, their prioritization process shouldn’t differ dramatically.
Always look for the applications of AI that align with your organization’s business strategy, offer the most ROI and pose the least amount of risk. Choosing how to invest in AI is really a question of how to best leverage its speed and personalization to make a superior and more specific product.
Speed means orchestrating thousands of machines—large-scale infrastructure—to perform a task or operation in parallel. Identify a security, development, or language issue, and find out how AI can solve it better and faster. To create a more personalized product with unique solutions, tailor your AI product so it can be trained off both public information and proprietary data.
The companies successfully implementing AI have simply optimized how they identify risks, close those gaps, and learn from customer behavior. In advertising, for example, manual testing of single ads has been surpassed by machine learning that tests many ads targeting different types of customers simultaneously. Now generative AI is doing all of that unsupervised, plus parsing vast amounts of data in real time for advanced analytics.
Measuring the maturity of processes in an organization is a good frame of reference for self-assessment. How far along is your company in terms of allowing the tech to run unsupervised? Historically, we have used supervised technology, but it can be slow and cumbersome.
But as machine learning and deep learning create greater footprints, people are increasingly getting out of the way—to their own advantage.
2. Gradually Move From Supervised To Unsupervised
The major point of difference with implementing AI over more traditional process efficiencies is the ethical or regulatory landscape. The capacity of machine learning for autonomous learning and decision-making can leave businesses exposed to unintended consequences if they let things get too far out of their control.
A proper risk assessment should address compliance and data security, as well ensuring training sets do not include bias and inaccuracies. Once businesses have the guardrails of clean data in place, they can begin testing and piloting AI. Start small in a controlled environment, such as a specific department. The model can be refined based on feedback and benchmarked against existing processes.
Bear in mind that the enterprise use case of AI is not going to come fully online tomorrow. Finding third-party partners to embed best practices and conduct regular inspections can help avoid unnecessary risk and strike the right balance between supervised and unsupervised use of AI.
3. Prepare The Way For A Cultural Shift
When the internet redefined retail, brick-and-mortar stores were not supplanted by e-commerce companies. With consumers now expecting a mix of digital and physical interactions, automation has allowed staff to be redeployed in value-adding roles. In a similar way, if AI is going to be a core pillar of the structure leaders are building, then they must learn to trust the technology and engage employees as willing participants in the transition.
I suggest leaders get familiar with AI by using it day to day. This can break down barriers to allowing it to offer more contextualized insights rather than trusting it only for raw data. For employees, training and upskilling are crucial components of an incentives program that rewards people for their adaptability and helps allay fears about job losses.
While businesses will no longer need an army of people to do some of the more mundane tasks, the efficiencies introduced by AI are going to create more opportunities elsewhere. Automation allows a broader range of people to get involved in specialized tasks, such as fraud detection, that used to be the exclusive domain of top experts.
It’s an assurance worth reiterating to employees wary of AI impacting their livelihoods: The aperture for the domains in which people can work is being widened, not tightened.
Building Agility Into The Future
Deep learning uses language models to mimic how humans think and communicate—and generative AI is the interface.
While the hype over AI delivering more human-like content is real, most business leaders would do well not to get distracted. Developments in this space are not cinched up and done, so adaptability is a key attribute as we move forward. When leaders and employees alike understand that AI enriches the way companies are doing business, they can use the tech to build an organization’s resilience and agility for a future we’ll all share.